New Drug AI-Screening Technology Based on Mitochondrial Phenotypes Developed by Teams of CPS-ZJU
2024-09-15 | 药学院英文网
Recently, the Yiyu Cheng/Yi Wang team from College of Pharmaceutical Sciences, Zhejiang University, in collaboration with a team from Stanford University, proposed a new deep learning method, MitoReID, for automatically learning large-scale data on cellular morphology, and by constructing the world's first large-scale dataset on mitochondrial dynamics under the action of drugs (more than 570,000 single-cell morphology images), the team found that the targets of FDA-approved marketed drugs have correlation to mitochondrial phenotypes, thus the targets can be inferred based on high-content screening images, which is a breakthrough in the technology of intelligently recognising the drug targets from the cellular screening model, and then invented an AI screening technology of new drugs based on mitochondrial phenotypes. The related research results were published on Nature Computational Science (Nat Comput Sci, 2024, 4:600-614). The paper was highlighted and introduced on the section Drug Discovery of the Nature website under the title of "AI-Recognized Mitochondrial Phenotype Enables Identification of Drug Targets"(https://www.nature.com/subjects/drug-discovery). In a concurrently published Research Briefing, senior editor Kaitlin McCardle commented that, "This approach has the potential to improve drug design by enabling a better understanding of drug MOAs."
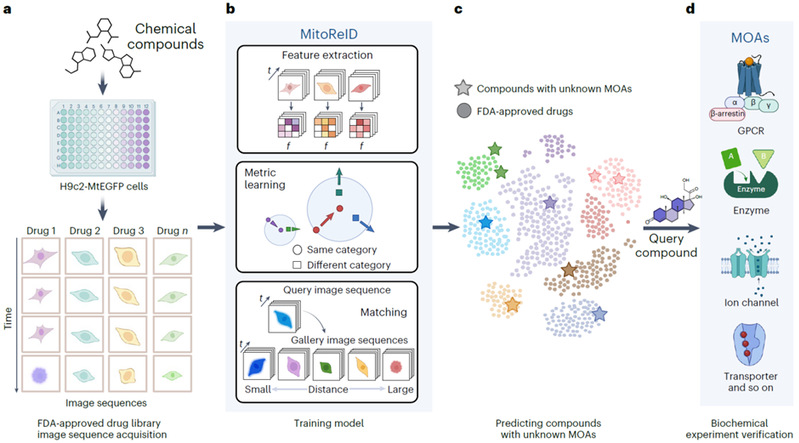
Drug target identification is a key aspect of new drug discovery. Although several chemical biology methods have been used to analyse the Mechanism of Action (MOA) of active compounds, there is still a lack of low-cost and simple methods for large-scale target identification. In recent years, image-based phenotyping methods have been emerging to identify drug targets by extracting useful information from biological images. However, in the face of the time-space nuances of cellular morphology and subcellular structure, there is still a huge technical challenge in how to effectively extract the multi-dimensional features in high-throughput screening datasets. To this end, the research team developed the MitoReID method for mitochondrial dynamic phenotype identification based on the Person Re-identification (ReID) algorithm, which automatically extracts the robust image features of individual cellular mitochondria in the process of temporal changes, and predicts the potential targets of unknown compounds by means of large-scale self-supervised pre-training and time dimension enhancement, providing a novel tool for AI screening of new drugs and new use of old drugs based on large-scale cellular phenotypic data.
The team firstly tagged the mitochondria of H9c2 cardiomyocytes with green fluorescent protein to establish a high-content screening method for mitochondrial morphology and membrane potential; and constructed a large-scale dataset of cellular mitochondrial dynamics by collecting temporal images of mitochondrial phenotypes of more than 1000 FDA-approved and marketed drugs after their effects. The established MitoReID deep learning model adopts a re-identification framework, and is self-supervised pre-trained by a time dimension enhancement method, and subsequently fine-tuned on a drug-target dataset covering more than 30 different classes of mechanisms of action, which can be queried to match the feature similarity between new drugs and target-known drugs in the training set to intelligently infer potential targets of action.
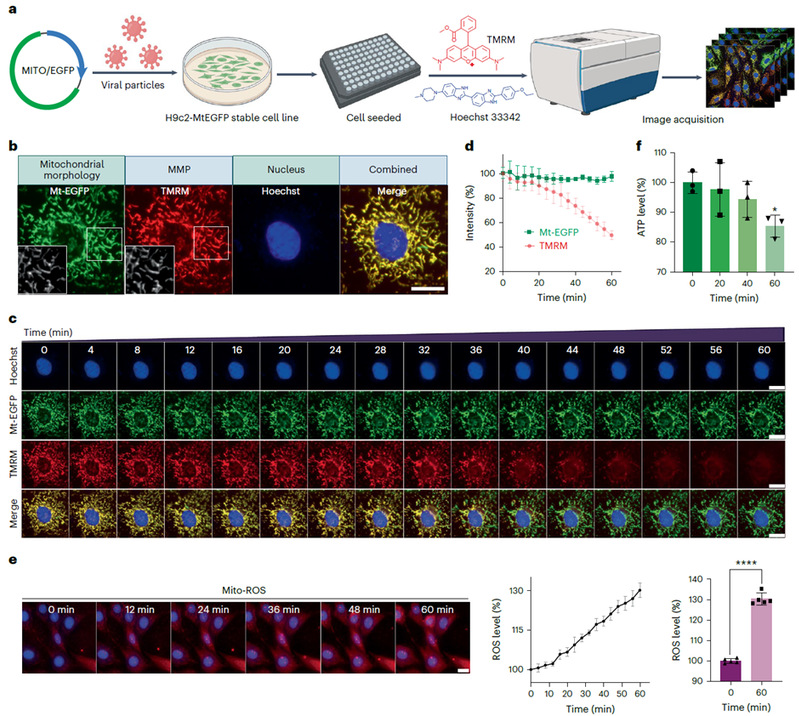
Experimental results indicate that MitoReID outperforms conventional machine learning algorithms in the test set, achieving 76.3% rank-1 accuracy and 65.9% mean average precision (mAP). In addition, MitoReID successfully identified six new drug targets that were not included in the training set. The model has also been extended to the discovery of pharmacodynamic substances in traditional Chinese medicine, predicting the potential targets of 60 important active ingredients in herbal compounds or herbs such as Scutellaria baicalensis, and discovering that epicatechin, a natural flavanol compound, may have the effect of inhibiting the enzyme Cyclooxygenase (COX). In vitro experiments further verified that epicatechin directly binds to COX-2 protein and significantly inhibits COX-2 activity.
This study demonstrates that analysing the dynamic effects of drugs on cellular mitochondrial morphology by AI technology can improve the understanding of the mechanism of action of new drug candidates, and can also lead to the discovery of potential targets of active ingredients in traditional Chinese medicines at a lower cost. This method provides a new idea for integrating multimodal phenomics data for drug discovery, which not only provides important technical support for the new use of old drugs, but also provides a new model for the application of AI technology to accelerate scientific discovery.
Doctoral student Min Yu from College of Pharmaceutical Sciences, Zhejiang University and Weiming Li from Zhijiang Laboratory are the first authors. Prof. Yi Wang and Prof. Yiyu Cheng from CPS-ZJU and doctor Zhang Xingcai from Stanford University are the co-corresponding authors.
Translator: Zihao Liu
Editor: Yichen Zhu
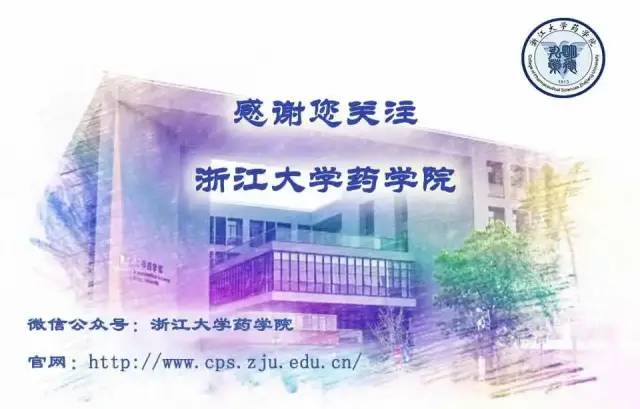
Discovery
-
18
2024.09
-
15
2024.09
-
15
2024.09
-
15
2024.09
-
15
2024.09
-
31
2024.08