EaslFA, an enzyme catalytic site annotation model based on multimodal deep learnig tecnology designed by research team from CPS-ZJU
2024-08-31 | 药学院英文网
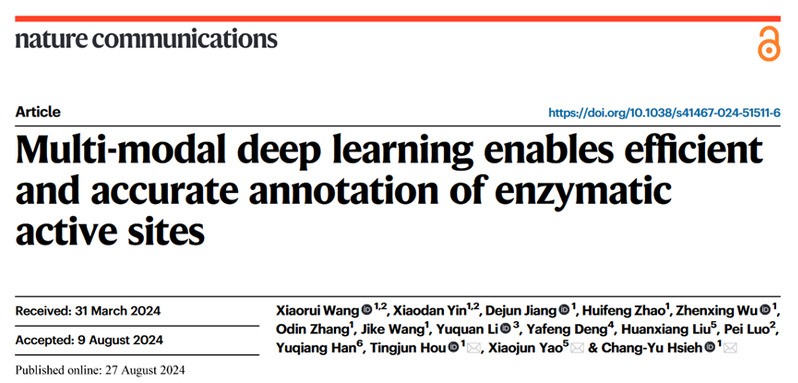
On 27 August 2024, the team of Changyu Xie and Tingjun Hou from College of Pharmaceutical Sciences, Zhejiang University, and the team of Xiaojun Yao from the Macao Polytechnic University published a research result titled "Multi-Modal Deep Learning Enables Efficient and Accurate Annotation of Enzymatic Active Sites" on Nature Communications, proposing a multimodal technology-based enzyme catalytic site annotation model, EasIFA, which incorporates potential enzyme representations from a protein language model and a 3D structure encoder, and then uses the multimodal cross-attention framework to combine protein-level information with knowledge of the enzyme reaction for more efficient and accurate prediction of the enzyme's catalytically active site. Compared with other similar methods that are currently optimal, EasIFA achieves at least a 10-fold improvement in recognition speed and at least a 9.68% improvement in active site recognition quality (measured in F1 scores) compared to conventional algorithms, and achieves about 1,300-fold speedups relative to high-precision deep-learning algorithms based on Position Specific Score Matrix (PSSMs) and GNNs, as well as supporting the recognition and detection of a greater variety of enzyme active sites.
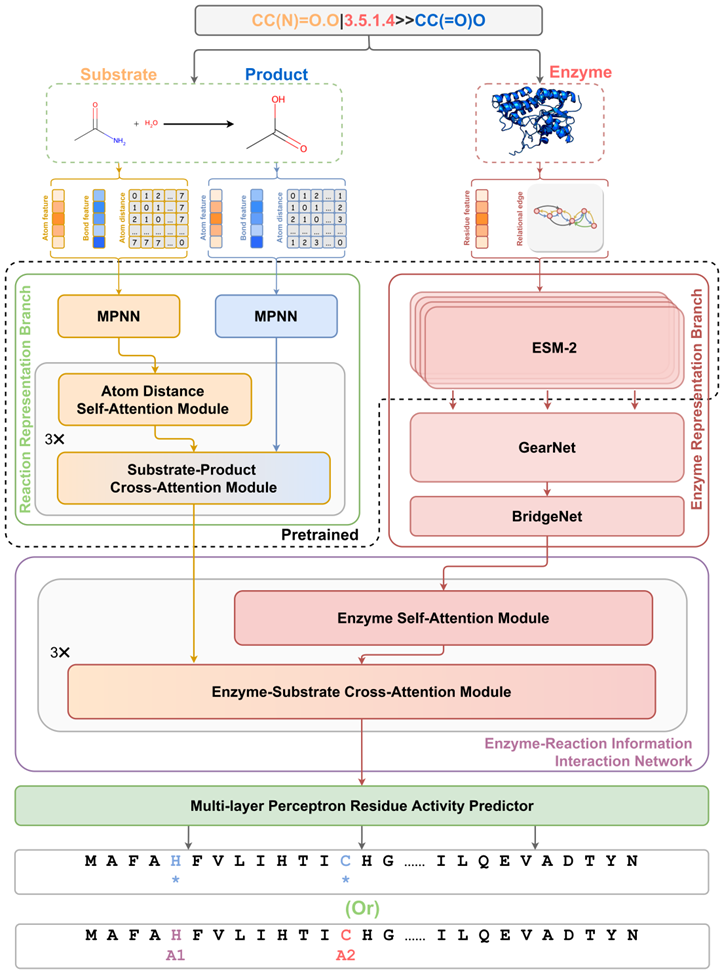
College of Pharmaceutical Sciences, Zhejiang University is the first signatory of this paper. Xiaorui Wang, a postdoc from CPS-ZJU, is the first author. Prof. Changyu Xie and Prof. Tingjun Hou from Zhejiang University, and Prof. Xiaojun Yao from Macao Polytechnic University are the co-corresponding authors.
Translator: Zihao Liu
Editor: Yichen Zhu
NEWS
-
28
2024.11
-
10
2024.10
-
16
2024.08
-
31
2024.08
-
26
2024.06
-
15
2024.06